The Pages of Tech History
A look at the evolution of technology from the pages of Computerworld
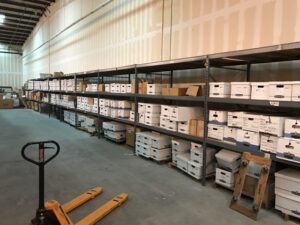
The Foundation has embarked on a storytelling project to share the legacy of Patrick J. McGovern’s visionary contributions to technology for good and to chronicle the evolution of how the digital transformation has benefited society. Our ongoing technology history project, led by MIT undergraduate Kathleen Esfahany, highlights milestones starting from the June 21, 1967 first issue of Computerworld, the flagship publication of Patrick J. McGovern’s technology publishing company, IDG. We will continue to share stories of tech development and innovation from the 1970s, 1980s and 1990s.
About this project
By Deborah O’Neil
BOSTON – In 1964, a young Patrick J. McGovern founded a small publishing company in his Newton, Mass home that would focus on the emergence of computer technology. Back then, computers were expensive, highly specialized and limited in use. Yet, the 27-year-old MIT graduate was years ahead of his time in envisioning the computer revolution, and he set out to document this transformation with the launch of his first magazine, Computerworld. His company, International Data Group or IDG, became a global publishing giant and Computerworld was its flagship publication, chronicling the history of computer technology.
More than fifty years after the launch of Computerworld, the Patrick J. McGovern Foundation has embarked on a storytelling project to share that history and trace the evolution of how computer technology has benefited society. The storytelling is led by fellow MIT Beaver Kathleen Esfahany, an undergraduate computer science major who remembers seeing Computerworld magazines around her house growing up.
The project began in 2017, when the Foundation worked with IDG to retrieve dozens of boxes of IDG print magazines stored in a Framingham, MA warehouse. Then, the Foundation arranged for all of the issues to be preserved by the world’s digital free library, the Internet Archive. A team of archivists housed in the Boston Public Library digitally scanned Computerworld and other print publications of IDG. The issues can be viewed and downloaded as PDFs at Archive.org.
As the Foundation’s Technology and Communications Intern, Kathleen mined issues of Computerworld and developing a storytelling series that highlights technology milestones, experimentations and policy. “Poring over the Computerworld archives gave me a newfound appreciation for how greatly technological innovation has transformed society. Computers have augmented everything from voting systems to medical testing. With each story I wrote, I found myself being transported back in time and feeling amazed at the impact of technology that we take for granted today.”
1990s: Growth of Personal Computers and World Wide Web
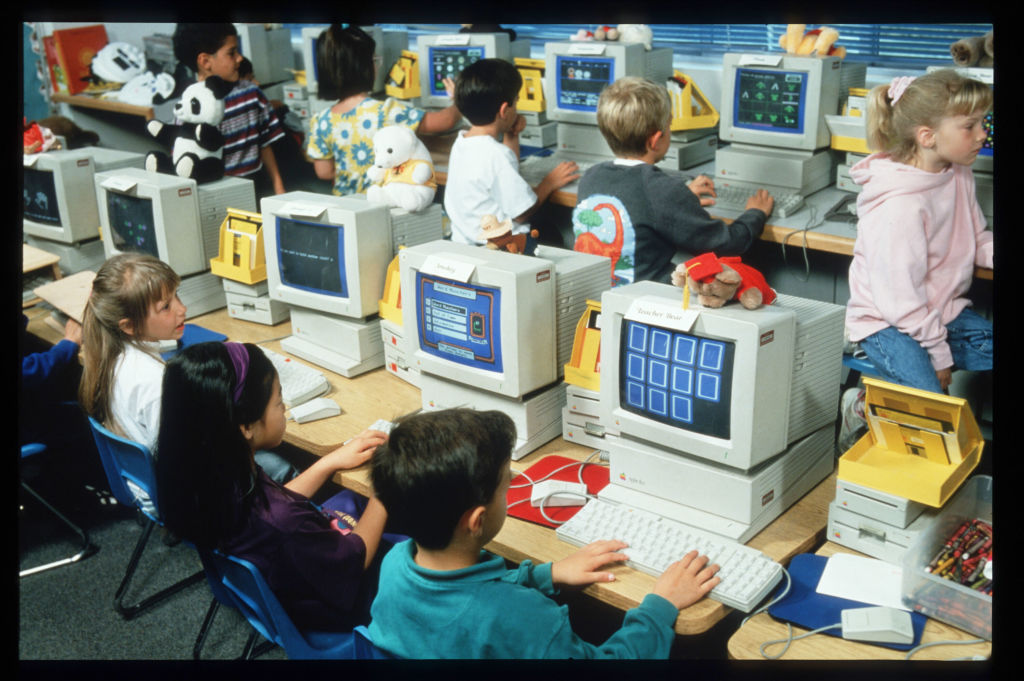
Feb. 5, 1990: MIT’s Project Athena advances distributed computing
May 3, 1993: Computer industry adopts green policies
May 3, 1993: Computer users overwhelmed by new “unstructured” information streams
Feb. 20, 1995: Videoconferencing gains popularity
Feb. 20, 1995: Massachusetts builds “motor voter” system
1980s: Rise of Data, Email and AI
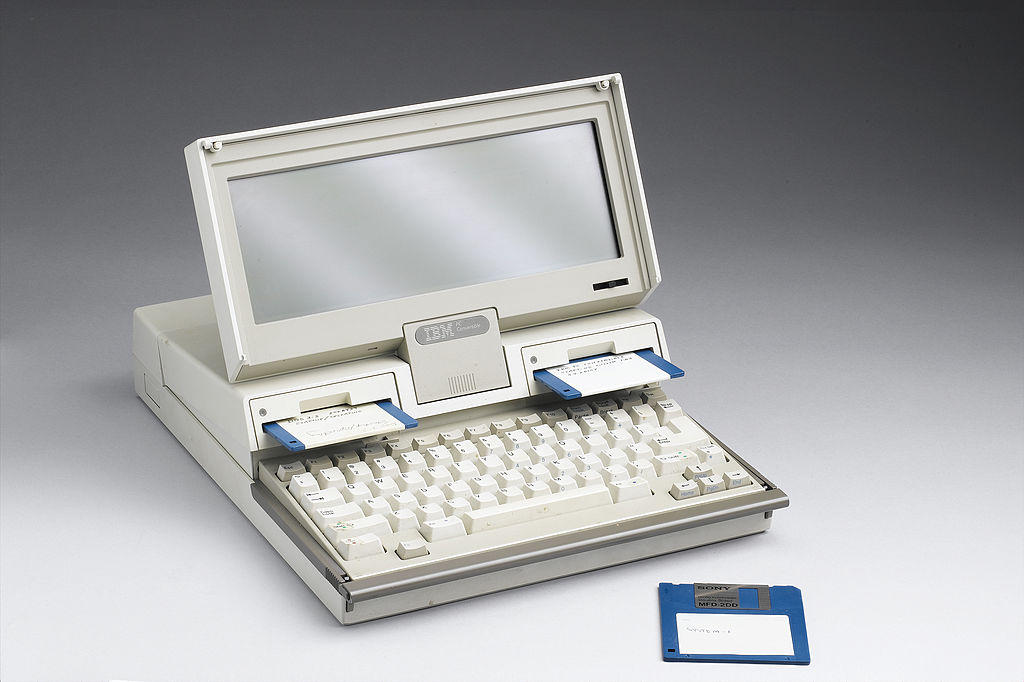
Jan. 30, 1984: Email recognized as important future business technology
Jan. 30, 1984: IRS streamlines operations with computer vision and digital storage
Feb. 16, 1987: SQL establishes dominance in database query language arena
Feb. 15, 1988: Neural networks generate interest and innovation in artificial intelligence
April 24, 1989: Museums automate collections management with custom data entry systems
1970s: Rapid Tech Advancements
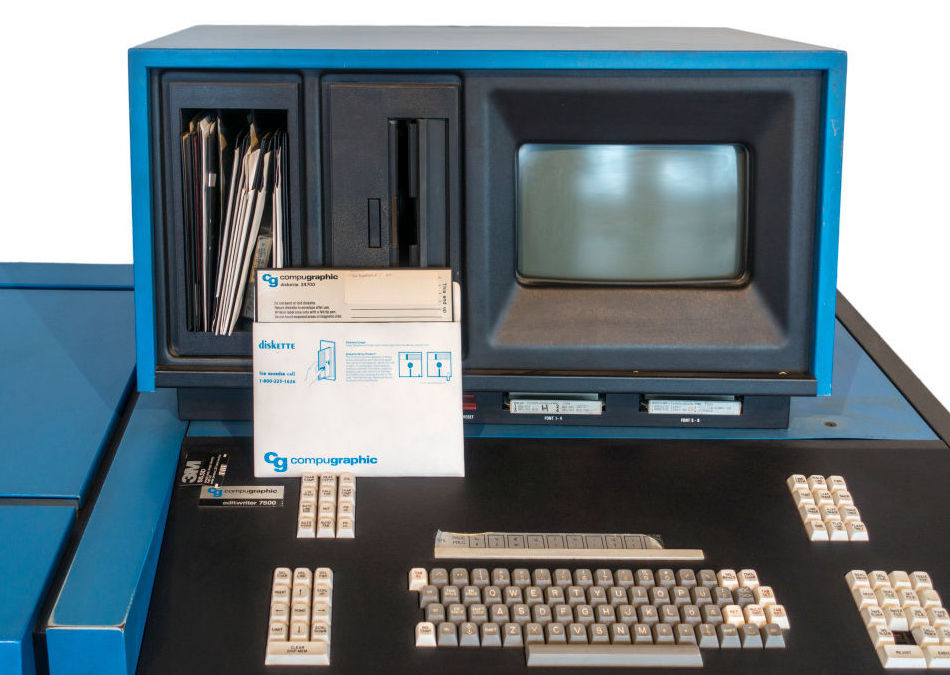
Feb. 2, 1972: MIT’s Project INTREX introduces computers to the library
Feb. 2: 1972: Chemists automate molecular drawings with computers
Dec. 20, 1972: UCLA student automates carpool system
Jan. 24, 1973: Computer optimizations reduce waste in furniture manufacturing
Jan. 31, 1973: House of Representatives casts first electronic vote
April 4, 1973: Accessible tools for computer users with visual impairments
April 4, 1973: Geologists use natural language system to study moon samples
April 11, 1973: Hawaii’s ALOHAnet system becomes the first satellite connection on ARPANET
1960s: Exploration & Experimentation
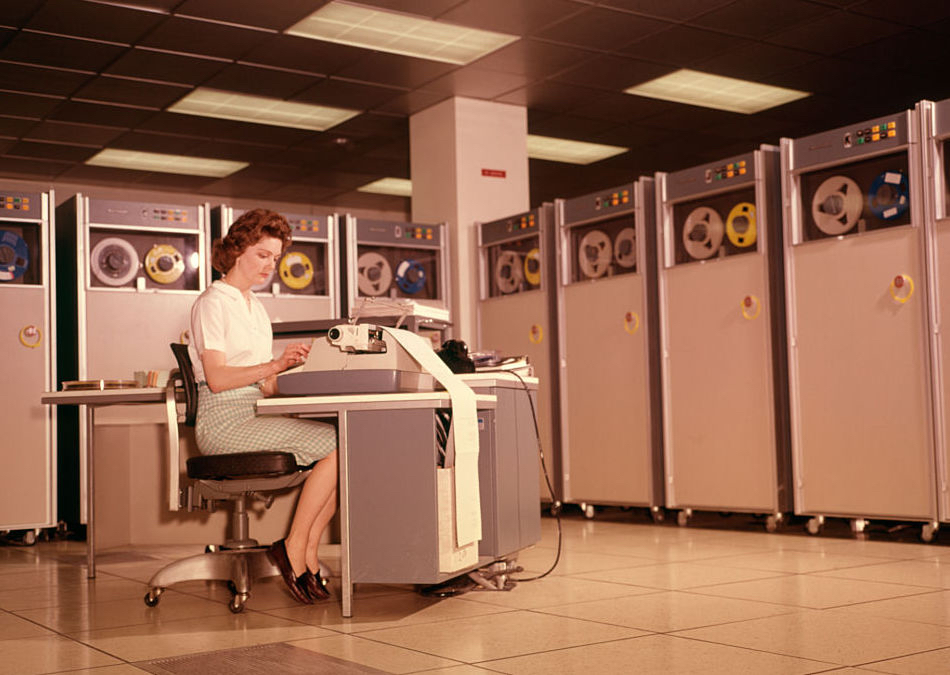
June 21, 1967: Computer languages COBOL and ADPAC revolutionize business world
Sept. 13, 1967: Americans file tax returns on magnetic tape
Oct. 11, 1967: Computer vision sees early breakthrough with “Electronic Retina” system
Nov. 1, 1967: Atomic Energy Commission’s 125 GB storage system breaks world record
Nov. 22, 1967: Analog computer simulations shake up agriculture
March 27, 1968: ASCII character encoding adopted as federal standard under President Johnson
April 3, 1968: Students rule ancient cities in early educational video games
April 3, 1968: Early visions for self-driving cars relied on computerized roadways
May 8, 1968: The Baseball Encyclopedia becomes first book with computer typesetting
June 19, 1968: First software patent awarded to Martin Goetz
June 26, 1968: Town attempts punch-card ballots and computer tabulation in 1968 elections
Dec. 4, 1968: Patentability of computer programs legally recognized in Prater and Wei
Jan. 15, 1969: Automated manufacturing transforms how post office box keys are made
Jan. 29, 1969: North Carolina installs computer system to track legislative bills
April 2, 1969: Michigan schools pioneer computerized attendance tracking
July 9, 1969: ACM hosts inaugural “Computer Music and Art Festival”
July 9, 1969: Computers lower cost and increase accuracy of eye exam
Sept. 17, 1969: Business recovers quickly after hurricane thanks to magnetic tape storage
Sept. 17, 1969: School bus route planning optimized by computer program
Oct. 1, 1969: Traffic bottlenecks relieved with automated transponder-based tolls