Ready to set up a data collaboration among nonprofits? Here are six keys toward success
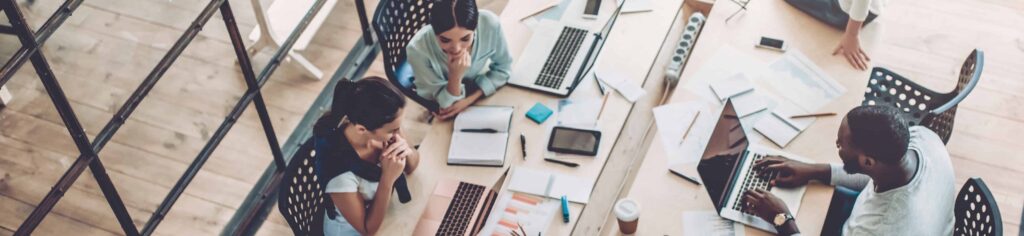
Editor’s Note: This article originally appeared on the Cloudera Foundation website. In April 2021, the Cloudera Foundation merged with the Patrick J. McGovern Foundation.
by Claudia Juech, Nikita Japra
Nov. 26, 2019
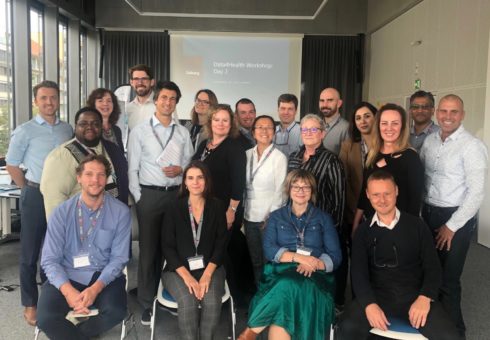
Many nonprofits collect, store, and analyze data, but very few give other nonprofits an opportunity to derive insights from those data. Even fewer purposefully collaborate to combine datasets and improve the group’s analytical capabilities.
In the field of digital health, for example, hundreds of bespoke applications funded by different entities aim to tackle either a particular disease or the health challenges of a specific population. Because these siloed projects often struggle to reach critical mass, they exist in a state of “pilotitis” — a disconnected series of efforts that fails to paint a full picture of a patient’s health or the strength of the health system as a whole.
Data collaborations among these digital health implementers could promote greater transparency and accountability, reduce redundancies in reporting, optimize resource allocation, and ultimately accelerate impact for organizations with shared objectives. And from a funder’s perspective, when such collaborations grow to include multiple data-driven projects within a given country, they become powerful enablers of governance — setting an example that provides the field with much-needed insights into best practices and performance benchmarks.
So what stands in the way?
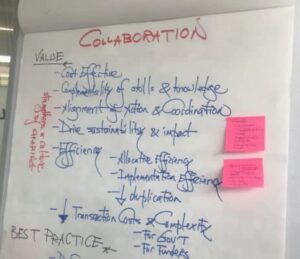
Managing data efficiently within one organization is a challenging task, and sharing it effectively and responsibly with other organizations can be even tougher. While the private sector has wrestled with data regulation and compliance even before the advent of the European Union’s General Data Protection Regulation (GDPR), many nonprofits are just beginning to explore technology-driven shifts in their practices across the data life cycle.
In the field of global health, this challenge is magnified by the need to protect sensitive data linked to the vulnerable populations that resource-constrained nonprofits tend to serve. And while development funders have increasingly called for data integration and collaboration among their grantees, the absence of established standards and best practices in the public sector has left many implementers to set the bar for responsible and effective data management practices themselves. This is especially true in countries where national data policies have yet to be fully implemented or regulated.
Without intentional alignment among program managers and data technologists across organizations, these nonprofits have yet to find a fit-for-purpose balance between what they can do and what they should do with their data.
The obstacles to nonprofit data collaboration are daunting, but they are not insurmountable. At the root of the challenge is the need to demonstrate a compelling case for why a collaborative approach is better than the status quo. In 2016, health and nutrition leads at Action Contre La Faim, Terre des hommes, and World Vision believed that the urgency of child survival could prove to be a financially and technologically sustainable use case for the practice of nonprofit data collaboration. A year later, they committed to a joint effort that would improve the integrated management of childhood illness and malnutrition using the latest advances in digital technology and data science. They called their alliance ALeDIA (Alliance for e-Diagnostics).
Bolstered by decades of combined experience in program design and development, the ALeDIA consortium knew that despite increasing investments in reducing child mortality rates worldwide, progress leading up to the Sustainable Development Goal era had been slow. The field of global health had already witnessed the launch of multiple pilot health programs that sought to leverage the latest digital technologies to improve the quality of diagnosis and care young patients could expect to receive at primary health clinics. Yet, so many of these promising pilots would fade into a fragmented digital health landscape just a few years after their initial launch — abandoned assets that would never see their way to scale without a dedicated funder.
At the same time, the consortium noted another troubling phenomenon — young children with signs of severe malnutrition were frequently turned away from health clinics because parallel, separately-funded nutrition programs only operated on site on a different day of the week. Like many of their peer organizations, the ALeDIA consortium was aware that multiple programs treating the same child rarely had the opportunity to compare notes or share data that could improve the child’s quality and continuity of care, let alone the child’s likelihood of survival.
“Coordination among decision makers across levels of the health system is critical,” Thierry Agagliate, Head of Innovation at Terre des hommes, explains, “but it is even more challenging when each nonprofit on the ground — whether focused on health, nutrition, or another vertical — has its own way of doing things, without a shared view of activities and information.”
The ALeDIA consortium’s alliance represents a new model of data collaboration — marked by the novel objective to accelerate improved health outcomes by linking data not only across levels of the health system, but also across sectors and implementing organizations. Successful implementation of such a model could set an example not only for the field of global health, but could also extend to other fields of human development such as education, the environment, conflict settings, and human rights.
In September, to further advance the development of this collaboration model, The University of Geneva and the Cloudera Foundation convened a cross-sectoral group of professionals to think through how to best collaborate across nonprofit organizations to share and integrate data for the greater good. The workshop was attended by digital health program implementers, data and tech specialists, policy-makers, researchers, strategy professionals, and funders — all of whom share a common goal of realizing the value of data to improve the health of communities around the world.
Here are six key takeaways from the workshop:
1. Data collaboration requires knowing yourself as well as your partners.
Siloed approaches to program implementation do not exist solely among nonprofits, but also within them. Before engaging with data collaborators, program leads should be familiar with their own organization’s stance on data privacy and security, common standards and data dictionaries, and practices across the data life cycle. Knowing these components for your own organization will help you determine how well-aligned existing policies and values across all your collaborators might be.
2. Data collaboration is a means to an end — but effective, responsible data management begins at the design stage of program development.
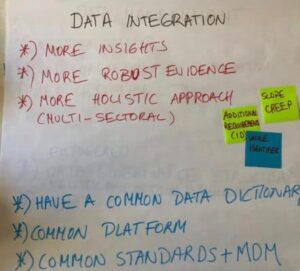
Often, program feasibility takes precedence at the planning stage, but it is not enough for collaborators to agree on a theory of change for program strategy. Workshop participants cited the benefit of conducting a theory of change exercise specifically focused on the value-add of collaboration, data integration, and shared data management practices across the program’s data life cycle. What is the problem each partner expects to solve through collaboration? For example — Will it accelerate impact? Improve research capabilities? Change standards of care? Each organization may have a different point of view on the benefits of data collaboration, and surfacing these differences in opinion at the start of a collaboration will ensure alignment on measures of success for the collaboration. It is imperative that program strategists, managers, and data technologists are all a part of this initial conversation.
3. Like any other collaboration, these efforts require a governance structure that is “fit for purpose” — striking a balance between what’s needed now and leaving enough flexibility to address the challenges to come.
To maximize the value of collaboration, clearly articulated roles and responsibilities among collaborators are critical to advancing work that depends upon limited resources and a rapidly evolving data ecosystem. Does one organization lead on data policy development? Does another offer a more advanced technology infrastructure? Who has the strongest relationship with key stakeholders, like the government and civil society? Allowing each organization to lead from its strengths not only maximizes efficiency — it may also make a more attractive investment case for funders.
4. Nonprofit organizations can help define data norms and standards in countries where the data policy landscape is more nascent.
Nonprofit organizations often collect data in policy vacuums, and can therefore lead the way on responsible behavior and policy support. As entities focused on protecting the rights of the most vulnerable, many nonprofits feel the ethical obligation to use and manage individuals’ data in a responsible way, but few entities have firmly defined what that means in practice. Defining the threshold of comfort with your collaborators early on is key, as there is often a fine balance between maximizing the immediate benefits and mitigating the long-term risks of data sharing. Successful use cases can set an example that others may choose to follow until the broader policy environment matures.
5. Dedicated funding for data collaboration is a necessity, not a luxury.
While internal buy-in is important to advance a nonprofit data collaborative, buy-in alone is not enough. Collaboration announcements too often dissolve when routine responsibilities compete with new priorities that don’t have a clear resource commitment from all partners. Such commitments should be explicit in terms of both time and money spent on staff time spent managing the collaboration process at all levels of the organization — from software developers and program managers, up through to CEOs and CIOs.
6. Just because we’re afraid of something doesn’t mean a solution doesn’t exist.
The pressure to ethically, responsibly, and effectively share, integrate, and manage data has a tendency to stunt program implementation — some would rather do nothing than introduce the potential for a privacy breach and its cascading consequences. Often the underlying assumption is that the tools and technologies we need to safeguard our beneficiaries TODAY as well as TOMORROW are still beyond reach, when they actually exist right now. Specific forms of encryption, user and view rights, and audit trails are examples of measures commonly deployed to protect beneficiary data. And where immediate solutions don’t yet exist, fit-for-purpose solutions can be built and adapted as programs launch and later mature. Data collaborators can benefit from conducting a workflow risk assessment — identifying privacy and ethics concerns at each stage of a program’s data lifecycle. With these risks clearly articulated early on, it is easier to determine whether and when they can be mitigated through new technologies or processes — without compromising impact.
Claudia Juech is the Vice President of the Data and Society program.
Nikita Japra is the Senior Manager of the Data and Society Accelerator Program.