Lessons to consider before we can deliver on the promise of data for impact
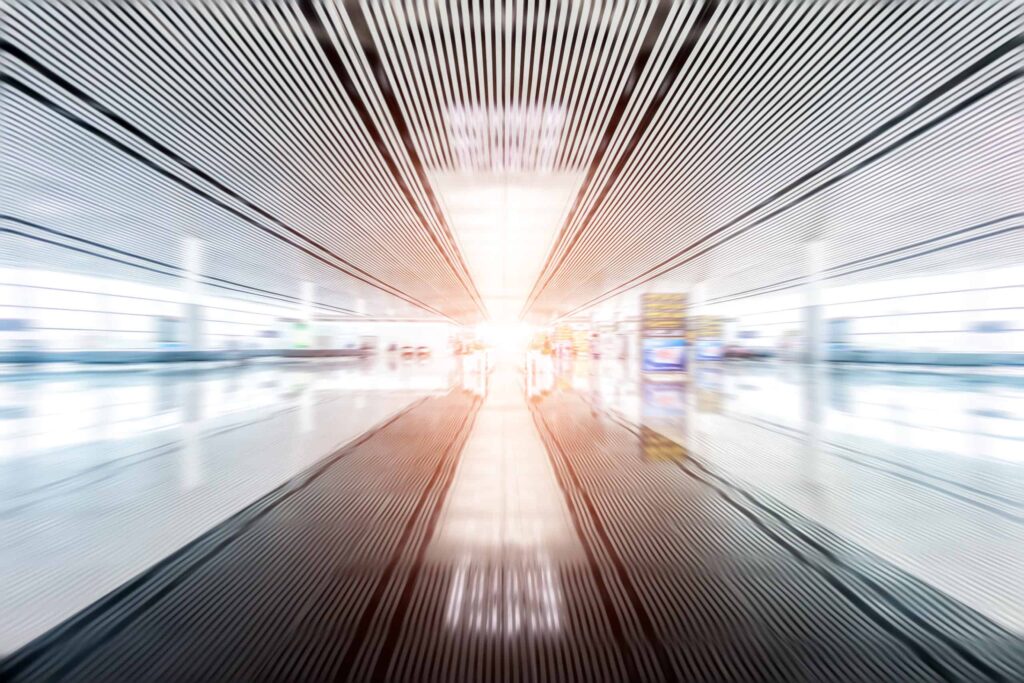
Editor’s Note: This article originally appeared on the Cloudera Foundation website. In April 2021, the Cloudera Foundation merged with the Patrick J. McGovern Foundation.
by Claudia Juech
Oct. 13, 2019
The promises and pitfalls of data can be told along two fictional storylines that could become a reality in the near future.
Story one. After multiple pilots, digital health tools now autocorrect the diagnosis and treatment decisions of nurses and community health workers in cases of pneumonia, malaria and childhood stunting. An independent impact evaluation confirms a 15% reduction in child mortality when those digital health tools are used in combination with an AI stethoscope and a child growth monitor app.
In story two, one of the largest nonprofits in the world is hit by a data breach that exposes sensitive medical information belonging to current and former beneficiaries, many of whom are children and members of a persecuted ethnic minority. As a consequence, the nonprofit loses many of its individual and corporate donors and has to close down several programs.
Two very different stories you might say, one the story of a successful AI intervention, the other the story of a data fail. In reality, however, both stories could be about the same nonprofit. And if we expand the time frame, we could tell the same story from even more angles. For example: Five years into the program, a second impact evaluation finds that the mortality rates for girls from a specific ethnic group did not decreased because of inherent biases in the data training set used to determine stunting.
In the data space, benefit and harm are invariably connected. So how can we reap the benefits and minimize harm? In the last two years I have spoken with many funders, experts and more than 300 nonprofits working on issues as different as wildlife poaching, human trafficking or financial inclusion. From those conversations, I would like to share three takeaways.
1. The use of data is not an easy solution to complex problems.
The end-to-end data use case is almost always as complex and messy as the problem. Having said that, I believe that the tech and data science requirements — while not easy — are actually the easiest pieces of the puzzle to solve. Data projects – aiming to solve societal challenges at scale – will always be complex because they require a multi-stakeholder approach involving the individual or group that’s facing the challenge, as well as government at multiple levels and other data holders.
Their governance is also complex not in the least because in many contexts governments have not yet established privacy and data ownership regulations. And they are complex because better information doesn’t necessarily lead to better action. Just as a good policy brief doesn’t necessarily make for better policies, we need to be clear how data insights will trigger action. When we at the Cloudera Foundation are doing theory of change exercises specifically focused on the data aspect of an organization’s work, it’s striking that it’s often hard to clearly articulate. why government officials, for example, would modify their behavior in light of new insights.
If we are using data to address complex problems I like a principle that I recently heard: Make it as simple as it can be, but not simpler.
2. There is more to AI than data collection and data science.
Many nonprofits come to us wanting to learn how they can turn their rich data assets into gold. Most of them can describe how they collect data, some also have a vision of what the data might be able to tell them. Even fewer have a sense of how to become a more data-driven organization where all aspects of the data lifecycle are purposefully being managed – from defining what to collect and for what purpose, to cleaning, quality control, storage, and reuse. Funders also often focus on supporting data collection or data analysis efforts with an implicit assumption that the steps in between will be taken care of — and in turn contribute to further pilotitis. The principle that I would like to suggest here: Understand and support the full data journey to reap the benefits and minimize harm.
3. We are bad at learning from successes and failures.
This statement is true for the development sector in general and maybe even more so for the data space. Although there pilots abound, it’s hard to find out why projects didn’t go into production. Did they truly fail or were they just not successful enough (i.e. their value add was not greater than the effort required)? Even for “successful” projects it’s difficult to get a clear picture of what constitutes success. In response — and because it has been proven to be difficult to scale individual projects — many funders now focus on creating frameworks, standards, repositories, and the like to generalize learnings and facilitate replication. That is useful. However, there is a middle layer between the project level and the meta level of a generic framework where the translation takes place, and this level doesn’t receive sufficient attention. Third takeaway: We need more transparency about what works and what doesn’t so that we can support the implementation of frameworks in specific contexts.
Claudia Juech is the Vice President of the Data and Society program.